Understanding the readiness of your source data before you launch an expensive AI/ML project lets you take corrective data engineering measures that will streamline the project and give you the best probability of a successful outcome. Artificial Intelligence (AI) and Machine Learning (ML) projects can provide significant returns on investment when they are applied to narrow but difficult business problems and are supported by adequate amounts of relevant, quality data. Many such projects start with high hopes but get derailed due to fundamental problems with source data, which were...
Joshua Powers
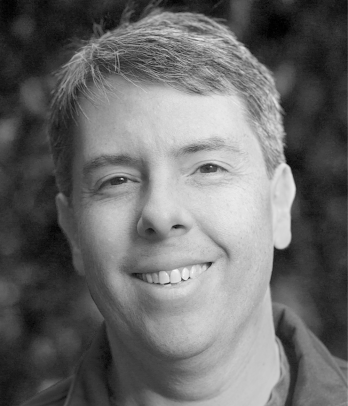
Joshua Powers is the Technical Director for AI/ML at Dev Technology Group. Josh has 30 years of experience researching and applying artificial intelligence technologies to various business and public sector challenges. Before Dev Tech, he was VP of Data Analytics at WorldAware. He developed a large-scale open-source intelligence exploitation platform to address geospatially and topically diverse intelligence requirements automatically. Before that, he was the CTO of LemonFish Technologies, a startup focused on data breach detection. He designed and led the development of their flagship platform, the LemonFish Trawler, creating innovative techniques for query by example, federated search, and semantic analysis of illicit Dark Web marketplaces. Josh has been a principal investigator for AFRL, DARPA, and IARPA projects. He has been awarded patents in semantic search, document clustering, and concept learning.